Mastering Data Visualization: 5 Best Practices for Clear and Impactful Insights
Data Visualization Demystified: A Comprehensive Guide to Creating Engaging Visuals that Uncover Hidden Insights.
M. Gibbs
2/17/20237 min read
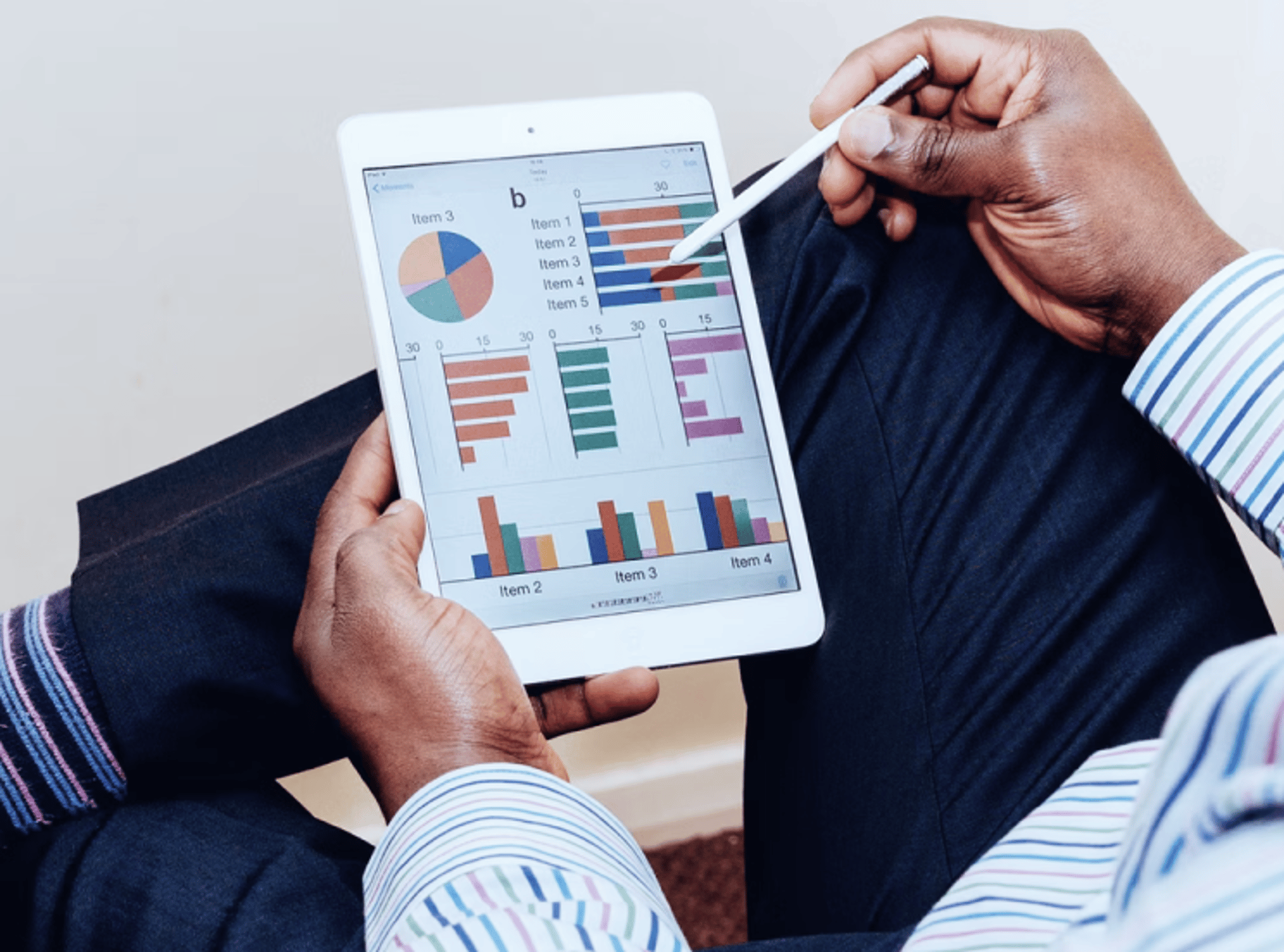
Data visualization is a powerful tool that enables us to unlock the potential of data by transforming complex information into clear, concise, and visually compelling insights. To ensure the effectiveness of your data visualizations, it's crucial to follow best practices that streamline communication and enhance understanding. In this article, we will delve into five key best practices for effective data visualization, supported by reputable sources and accompanied by real-life examples.
Simplify Complexity:
Complex data can overwhelm audiences, hindering comprehension and diluting the intended message. By simplifying complexity, you can distill the essence of your data visualization. Edward Tufte, a renowned expert in data visualization, advocates for data-ink ratio, suggesting that each element in a visualization should contribute to conveying information. For example: A line chart depicting stock market trends with minimal gridlines, clear axis labels, and a focus on the overall trend rather than excessive data points.
Consider these additional insights and techniques that can help to simplify complexity in data visualization:
Remove Non-Essential Details: Strip away any visual elements that do not contribute to the understanding of the data. This includes unnecessary gridlines, decorative elements, or excessive data points.
Use Clear and Concise Labels: Ensure that axis labels, titles, and annotations are concise and easy to comprehend. Avoid using jargon or overly technical terminology that might confuse the audience.
Emphasize Key Information: Identify the key insights or main messages you want to convey and give them prominence in your visualization. This can be achieved by using visual cues such as color, size, or positioning to draw attention to the most important elements.
Group and Categorize Data: When dealing with large datasets, consider grouping or categorizing the data to simplify the visualization. This can involve aggregating data into meaningful categories or using hierarchical structures to organize information.
Utilize Summary Statistics: Instead of displaying every data point, consider using summary statistics, such as averages, totals, or percentages, to provide a high-level overview. This helps to distill complex information into digestible insights.
Choose the Right Chart Types:
The appropriate selection of chart types can significantly impact the understanding and interpretation of data. Understanding the data attributes and relationships is crucial for choosing the most suitable chart type. Start by thoroughly understanding your data and the variables you want to visualize. Determine whether your data is categorical (divided into distinct groups) or continuous (numeric and measurable). Consider the relationship between variables you want to showcase. Are you comparing values, showing trends over time, exploring correlations, or examining distributions? Different chart types excel at visualizing specific types of relationships. For example, bar charts and column charts are ideal for comparing categorical data or showing rankings, bar charts (horizontal bars) and column charts (vertical bars) provide a clear visual representation of values. They work well when comparing multiple categories or subcategories side by side.
Highlight Key Insights:
Effective data visualizations focus on delivering key insights to the audience. By utilizing visual cues such as color, size, or annotations, you can highlight important findings and guide viewers' attention to the most critical aspects of the data. Some additional techniques and considerations for highlighting key insights:
Color Emphasis: Utilize color strategically to emphasize specific data points, trends, or significant findings. You can use contrasting colors or make important elements more vibrant to make them stand out.
Annotations and Callouts: Directly annotate your visualization with text, labels, or callout boxes to provide additional context or explanations for key insights. Annotations can draw attention to specific data points or highlight important observations.
Visual Cues: Incorporate visual cues such as arrows, lines, or icons to guide the viewer's attention towards critical areas or trends within the visualization. These cues can act as signposts, indicating where the key insights lie.
Highlighting and Filtering: If you have a complex dataset, consider implementing interactive features that allow users to highlight or filter specific portions of the data. This enables them to focus on the relevant details and extract key insights for themselves.
Comparative Emphasis: Use visual elements such as size, position, or color to create visual comparisons that highlight key insights. For example, you can make a specific bar or data point larger than others to emphasize its significance.
Storytelling Techniques: Organize your visualization in a way that tells a coherent narrative, leading the viewer's attention towards the key insights. This can involve starting with an attention-grabbing headline or structuring the visualization to build up to the most impactful findings.
Interactive Hover Effects: Incorporate hover effects that provide additional information or details when the user hovers over specific elements. This allows users to explore the visualization and discover key insights on their own.
Ensure Data Accuracy:
Maintaining data accuracy is paramount to establishing trust and credibility in your visualizations. Conducting rigorous data validation, verifying data sources, and performing quality checks are essential steps to ensure the integrity of your visualizations. Here are some additional details and best practices to help you maintain data accuracy:
Data Validation: Validate the accuracy and integrity of the data through various validation techniques. This involves performing checks for completeness, consistency, and conformity. Validate data against predefined rules, such as data type constraints, range checks, and logical validations.
Outlier Detection: Identify and handle outliers effectively to maintain data accuracy. Outliers are data points that deviate significantly from the expected patterns. Detecting outliers helps identify potential data entry errors, measurement issues, or anomalies that may impact the accuracy of the overall dataset.
Data Cleaning and Preprocessing: Prior to analysis and visualization, conduct thorough data cleaning and preprocessing. This includes handling missing values, standardizing formats, resolving inconsistencies, and removing duplicate records. Cleaning and preprocessing steps help eliminate data errors and ensure data accuracy.
Documentation: Document the data collection process, including sources, methods, and any transformations applied. Maintaining detailed documentation enables transparency, allows for reproducibility, and helps identify potential issues or biases that may impact data accuracy.
Data Governance: Establish data governance practices within your organization to ensure data accuracy and reliability. This involves defining roles, responsibilities, and processes for data management, including data quality checks, version control, and access controls.
Cross-Verification: Cross-verify data with multiple sources, if available, to ensure consistency and accuracy. This helps identify any discrepancies or outliers that may require further investigation.
Regular Auditing: Conduct regular audits of your data to identify and rectify any data accuracy issues. This includes performing periodic checks, reviewing data quality reports, and implementing corrective actions.
Collaboration with Domain Experts: Collaborate with subject matter experts or domain specialists who possess in-depth knowledge of the data and its context. Their expertise can provide valuable insights and help ensure data accuracy through thorough validation and interpretation.
Quality Assurance Processes: Implement quality assurance processes specific to data analysis and visualization. This involves peer reviews, double-checking calculations, and conducting thorough testing of data transformations and visualization outputs.
Continuous Monitoring: Establish mechanisms for continuous monitoring of data accuracy, especially when dealing with real-time or frequently updated datasets. Regularly monitor data quality metrics, identify trends, and address any issues promptly.
Design for the Audience:
Adapting your data visualizations to the intended audience is crucial for effective communication. Tailor your visualizations to their level of data literacy and familiarity with the subject matter, ensuring that the visualizations are easily understandable and engaging. For example: using simplified terminology, providing contextual explanations, and incorporating interactive elements to allow exploration for a more data-savvy audience. Consider these aspects when creating visualizations that effectively cater to your target audience:
Identify Audience Characteristics: Understand the demographics, background, and knowledge level of your audience. Consider their familiarity with the subject matter, their preferences, and any specific requirements they may have. This understanding will shape the design choices you make.
Define the Purpose: Clarify the purpose of your visualization and what you want to communicate to your audience. Are you aiming to inform, persuade, or educate? Clearly defining the purpose helps guide your design decisions and ensures that your visualizations align with the intended message.
Simplify Complexity: Simplify complex information by focusing on the key insights and main takeaways. Avoid overwhelming your audience with excessive detail or unnecessary elements. Use clear and concise labels, titles, and annotations to guide their understanding and highlight important aspects of the data.
Visual Hierarchy: Establish a clear visual hierarchy to guide the viewer's attention. Emphasize important elements by using size, color, contrast, and position. Use visual cues such as arrows or callouts to direct the audience's focus towards key insights or trends within the visualization.
Tailor the Level of Detail: Adjust the level of detail in your visualization based on your audience's needs. For experts or data-savvy individuals, you may provide more granular information and advanced visualizations. For general audiences or those with limited data literacy, focus on presenting the main findings and simplify the visual representation.
Choose Appropriate Chart Types: Select chart types that best represent the data and support the audience's comprehension. Consider the nature of the data, the relationships you want to showcase, and the story you aim to tell. Choose chart types that are familiar to your audience to facilitate their understanding.
Use Intuitive and Consistent Design: Create visualizations that are intuitive and easy to navigate. Use familiar icons, labels, and design patterns to reduce cognitive load. Maintain consistency in colors, fonts, and styles throughout your visualizations for a cohesive and professional look.
Test and Iterate: Gather feedback from your audience and conduct user testing to evaluate the effectiveness of your visualizations. Incorporate the feedback into the design process and iterate on your visualizations to enhance their clarity and impact.
Consider Accessibility: Ensure your visualizations are accessible to a diverse audience. Use appropriate color palettes for color-blind individuals, provide alternative text for images, and consider using data sonification or other alternative formats for individuals with visual impairments.
Tell a Compelling Story: Weave a narrative or story around your data to engage and captivate your audience. Frame your visualizations within a compelling context that resonates with their interests, challenges, or aspirations. Craft a storyline that guides them through the data and helps them make meaningful connections.
Conclusion:
By implementing these five best practices for effective data visualization—simplifying complexity, choosing the right chart types, highlighting key insights, ensuring data accuracy, and designing for the audience—you can elevate your data visualizations to new heights. Simplifying complexity ensures that your visuals are easily digestible, allowing your audience to grasp complex information effortlessly. Choosing the right chart types empowers you to represent your data accurately and emphasize the patterns and relationships that matter most. Highlighting key insights enables you to draw attention to the most impactful findings and guide your audience's focus. Ensuring data accuracy builds trust and confidence in your visualizations, allowing for informed decision-making. Finally, designing for the audience ensures that your visualizations resonate with their needs, preferences, and level of data literacy. By embracing these best practices, supported by expert sources and real-life examples, you will create compelling visualizations that not only captivate and inspire understanding but also drive meaningful insights and actions.
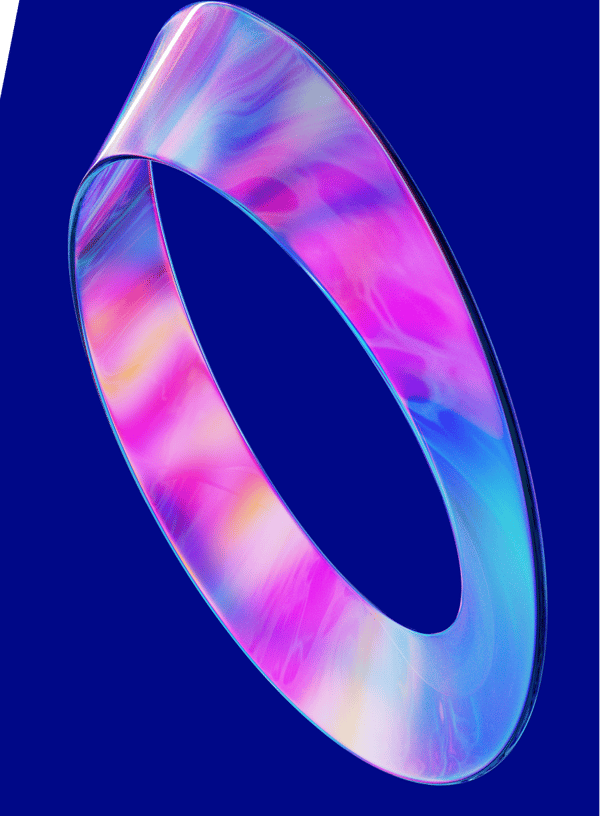

Contact us
Whether you have a request, a query, or want to work with us, use the form below to get in touch with our team.